Discovering Fake Consumer Reviews With GSRank
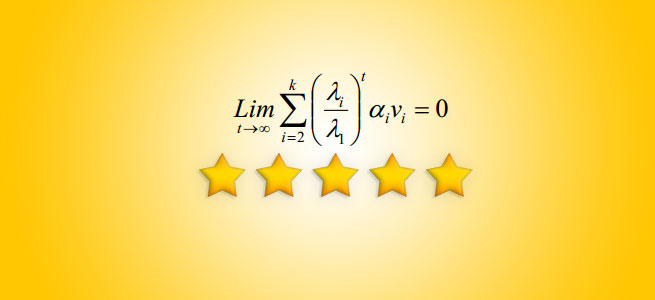
Researchers at University of Illinois at Chicago and Google have just introduced a new spam-fighting metric called GSRank which is a product of behavioural observation of spammer groups. The model which outperforms all existing spam fighting methods includes relationships between groups, individual activities and also observes reviewed products. It appears that flagging groups of product review spammers is easier than labelling them individually:
Basic Pattern of Fake Reviews
Observing a small group of three fake reviewers/accounts lead to the following findings:
- Same product reviewed by the common group.
- High rating by group members.
- Narrow time window within all rated products.
- Group reviewed the same set of products.
- Quick to rate. Usually first or among first to review.
The first step in product review analysis is to build a reference dataset. This involves data mining followed by labelling and defining group spam behaviour indicators. Group and individual behavioural statistics are examined and ranking experiments are conducted to train the system. The evaluated results are not only fast but also highly accurate.
Practical Application
This research don’t only help stores (Amazon) and their customers but it also carries much wider potential. Search engines can now apply GSRank model to discover fake reviews and normalise their aggregate ratings for products, places and various other features.
References
[1] Mukherjee, A., Liu, B., Glance, N., – Spotting Fake Reviewer Groups in Consumer Reviews, WWW 2012, April 16–20, 2012, Lyon, France.
Ivanovic J (2012-04-24 06:29:25). Discovering Fake Consumer Reviews With GSRank. Australian Science. Retrieved: Jun 30, 2025, from https://ozscience.com/internet-2/discovering-fake-consumer-reviews-with-gsrank/